Big Data and Big Pharma: A Case for Machine Learning
Sophia Booke discusses how big pharma can use machine learning to predict patient response, manage an excess of data and improve drug design
Add bookmark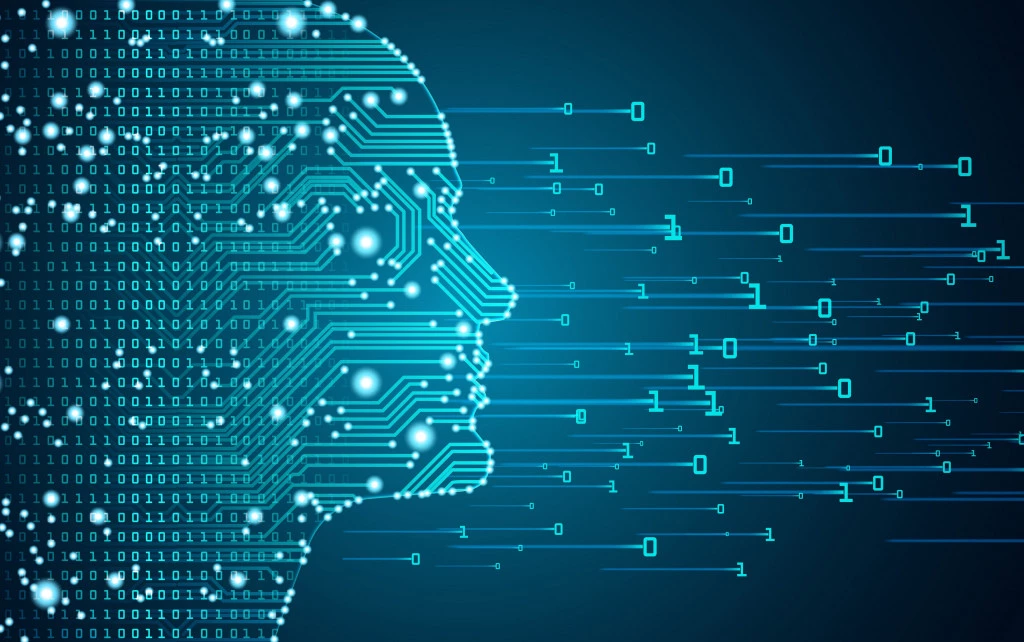
The long and costly drug development process made pharmaceutical companies think about new ways to upgrade their current business model. It looks like the pharma industry is on the brink of crisis right now. There are no more low-hanging fruit when it comes to drug development. Bringing a new product to market can take up to 15 years and cost over a billion dollars.
A new paradigm is necessary, and machine learning (ML) might be just the right solution. This is because it can simulate millions of tests taking into consideration multiple variables in a matter of hours and only highlight that worth pursuing.
Less trial and error
Until now, drug development included a great deal of trial and error work, sometimes with fatal results, and other times with unwanted delays. Machine learning models can offer another way of creating new drugs, starting with the patient and the problem and working backward.
Based on the significant advancements in genetics and genomics, machine learning can now offer the opportunity to create particular drugs by predicting the patient’s response to the treatment. Such models will take into consideration all the necessary parameters such as the history, allergies and other data.
What are the applications and examples of ML for pharma?
There are at least three major applications for machine learning in the pharmaceutical industry. We will focus on each, discussing pros and specific challenges.
Read the report to learn the importance of using data to create scientific value and how to digitize data for more efficient lab work. Download the Report.Pharma IQ Special Report: Unlock the Power of Data to Advance Pharma Businesses
-
Drug design, development, and testing
ML developers from InData Labs claim that through ML, pharmaceutical companies can create new drugs by exploring which molecules have a higher probability of binding together by testing millions of combinations at an atomic level. Starting with a target molecule and a library of known interactions, the system examines a wide array of possible substances. This idea has already been tested by Atomwise, IBM and Toronto University to try and find a cure for the Ebola virus.
ML can also be useful in finding new biomarkers to track diseases or to test for certain conditions. Not only it is helpful in the upper segment of the R&D funnel—in selecting the most likely substance candidate—but also further down. For example, it can help reduce animal and human trials by further refining the targets.
-
Predicting patient response
The drug design paradigm until now included creating substances which would solve classes of problems. It was the doctor’s duty to assign a patient to a particular disease class and come up with the treatment. Personalized medicine is reshaping this mode of thinking by working with reverse engineering to predict how a particular patient will respond to the procedure.
Based on the patient’s HER, the algorithms simulate how an organism will interact with a wide range of substances and design the most efficient drug. It is a matter of running thousands of combinations until the perfect match is found. This is called personalized medicine.
Most likely, it will become the medicine of the future and also provide the pharmaceutical industry with a new business model that is based on designing personalized health solutions.
-
Processing heaps of data
All ML models require vast amounts of clean and tagged data. Current records are stored both in digital and physical formats, most of the times in databases or silos which don’t communicate with each other. That’s why such AI systems should be able to connect multiple data sources into a centralized processing unit.
To be useful, ML needs streamlined data which is properly categorized and classified. Furthermore, it should be able to operate with various data types, from DNA sequencing to lab test results and general chemical interactions as well as allergies.
A Case in Point
HBR describes the case of a company which studies the triggers and situations when patients need to transition to another line of therapy. During this process, they uncovered three replicable steps which could act as guidelines for other pharmaceutical companies.
The first one is getting organizational buy-in by engaging the right stakeholders from multiple teams. The combined knowledge can generate a hypothesis to be tested by the computer.
The next concern is data, which is used to train and calibrate the system. In pharma models, the best data is, in fact, a combination of data sets. EHR is a good starting point, but external data coming from previous studies can be useful too. All records are then sent to an automatic feature discovery machine.
The last step is using feedback loops over and over again until results are relevant and replicable. This is necessary to remove noise and variation.
42% of the industry claim efficient lab data management will have the biggest impact on industry growth in the next five years. Find out how to get ahead of the curve. Download the Pharma IQ Report Now.Achieve Digital Business Success in Pharma with Data-Driven Approaches
What are the challenges in using ML for pharma?
There are significant obstacles to adopting machine learning for pharmaceutical development. The first barrier links to data. Such models require massive amounts of correctly labeled data to learn from. Right now, there are very few labeled libraries, and most projects start from scratch and require laborious manual work at this stage.
Another problem is related to the “black box” feature of machine learning. It is difficult to retrace the connections made by the hidden neural layers and correct any mistakes.
Last but not least, there is the challenge posed by the lack of relevant expertise. Until now, computer science, AI and drug development were different jobs involving experts with different academic backgrounds. To run a successful ML project though, they have to cooperate and learn from each other to create cross-functional teams.
What are the future expectations for ML in pharma?
Although numerous companies provide ML services for the pharma industry at the moment, some of these are not very transparent about the way their algorithms work. Others might rely on the results of a few studies they have done with positive outcomes.
However, as the underlying technologies advance, we can expect a sharp increase in the adoption of these techniques and, by extension, of a more patient-centered approach.