Optimizing pharmacovigilance processes with the latest technologies and practices
Sanyam Gandhi, Regulatory Strategy Lead at Takeda, analyzes how pharma organizations can achieve a future-state of pharmacovigilance through automation technologies
Add bookmark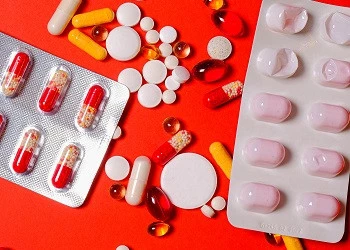
Pharmacovigilance (PV) is a vital component of the drug development process and can also be referenced to as PhV or drug safety. It protects the health and safety of healthcare consumers and keeps pharma manufacturers informed of any adverse drug reactions (ADRs) their products may cause in specific individuals. PV involves identifying, tracking, evaluating and preventing negative outcomes from drug therapies.
The PV market surpassed US$4.3bn in value in 2018, as per a Global Market Insight report which predicted the market would grow further to $8.9bn by 2025. The reason behind this projected growth is due to existing inventory of drugs as well as increases in the number of new drugs in development.
All of these drugs have to meet requirements and prove efficacy and safety to the US Food and Drug Administration (FDA) and similar regulatory agencies across the world before entering the market.
Automation in PV
Artificial intelligence (AI), robotic process automation (RPA) and machine learning (ML) are becoming increasingly prevalent in all aspects of our daily lives. These advanced technologies have the potential to significantly impact the way that PV operations are performed through individual adverse event (AE) processing powered by data analytics, signal detection and predictive analysis.
What is the reasoning behind using automation in PV?
During clinical trials or post-approval, the primary source of patient erosion is unpredictable toxicities that can cause morbidity and mortality from the normal dose of the drug. From 2008 to 2017, the FDA approved 321 novel drugs, as well as reporting more than 10 million AEs, of which 5.8 million were recorded as serious, and 1.1 million were death reports.
Additionally, AE reporting is significantly increasing year-on-year. The approval of new therapies, initiation of patient support programs that increase the level of patient participation, new regulatory requirements and an increase in the sources of AE reports – including social media and mobile medical applications – are all contributing factors to the increase.
ADR data needs to be collected by the license holder of a pharma company and submitted to the local drug regulatory authority. The most important operations in the PV industry are detection and reporting of ADRs, coding of AE in technical terms, preparing individual safety reports, assessment of seriousness and determining a relationship with the suspected drug. All of these operations depend on human interference, which is time-consuming, suggesting that the detection of ADRs requires new technology.
The cost of PV rising in both finances and resources is continually due to increasing numbers of incoming ADR reports captured by ongoing post-marketing surveillance (PMS) monitoring. Several factors, such as an aging population, increased health awareness among the public, and the number of pharma products on the market increase the amount of ADRs. When this is combined with the increased regulatory requirements over the past few years, it is easy to see how the costs of PV have ballooned for pharma companies.
A recent Deloitte survey of mid-to large-cap global biopharma companies shows that PV projects are increasingly looking to improve efficiencies while cutting costs. According to the study, cutting case processing costs was the primary goal of 90 percent of respondents. The survey also showed that many companies are investing in AI and automation to tackle various PMS-related tasks. After all, the sheer volume of healthcare and other data available for analysis makes it practically impossible for humans to track and analyze this manually and efficiently.
If any organization is not able to efficiently manage, detect and process AEs in a timely manner, they may have to face revenue loss from financial penalties and product recall. Automation will help with quick and efficient handling of high volumes of AEs.
What are the opportunities of automation in PV?
Opportunities for automation exist across all PV processes, however, the best example is the Individual Case Safety Report (ICSR) because of the high costs involved, the huge workforce, high risk of quality-related issues and operational inefficiencies. The generic ICSR process starts with the receipt of an AE report and is mainly grouped into three process blocks (see Figure one).
Figure one: The three process flow blocks of the ICSR process which are sequential as mentioned in above diagram
- Case intake: Duplicate check, prioritization, triage and local structuring steps can be considered major and favorable targets for automation. Triage and local structuring steps pose high risk for automation, however.
- Case processing: Full data entry and medical assessment are time consuming, complicated and high-cost tasks which also carry a high risk of quality issues. While most organizations are already applying some automation as part of narrative writing, labeling and prioritization of reports, the level of manual intervention remains high.
- Case reporting: Several steps, such as submission, distribution and acknowledgment are already automated, although the reconciliation step can benefit from further automation. Submission rules configuration and maintenance are not part of the core ICSR process, but are critical business configuration steps that enable automation of submission generation and distribution. These tasks are performed periodically or as needed and require high effort. The perceived risk for automating this process, however, is high because of the direct impact on regulatory reporting compliance.
Traditional drug safety and ancillary systems have provided incremental innovation and limited automation, but the burden of manually processing AE reports remains high. The advent of RPA, cognitive computing, AI and ML presents a significant opportunity to automate many of the manual PV processes.
What are the benefits of automation in PV?
- The most important benefits of automation are reduced training and processing costs and saving time through continuous processing.
- Global harmonization and simplification of data intake and processing.
- Improved speed, quality, and accuracy of information.
- Increasing case volume will not increase company headcount.
- Automation can handle diverse types of incoming data formats.
- Automation can assist with the identification of ADRs.
- Decreased compliance risk from missing AEs.
- Automation will overcome language barriers.
- Automation is useful to reduce the burden and increase the speed of case processing.
- Automation tools extract information from the ADE form and evaluate the case validity without the need for human involvement.
How AI is applied in PV?
Prevalent AI technologies in PV automation are ML, natural language processing (NLP), natural language generation (NLG) and RPA, among others. The following is a summary of how these technologies are applied in PV:
- Unlike human analysts, these tools can quickly identify, collect and analyze massive amounts of data. Because free-form text data is prevalent in healthcare, ML and trained NLP algorithms can detect, extract and classify ADR data from this kind of unstructured data.
- The greatest potential for the application of AI in PV comes with the automation of manual, repetitive, routine tasks with case processing. AI will decrease the cost of processing each case and free up valuable resources to work on more complex and value-adding tasks.
- Big data analytics using AI can also help discover drug-event associations for certain groups of people, improving the detection of potential events while improving risk-benefit assessments. Some estimate that AI has reduced screening costs by 80 percent and general annotation costs by 50 percent while eliminating setup costs.
- NLP algorithms can quickly analyze big datasets from social media sources, news articles, medical literature, medical records and other text data. This approach uses AI and trained analysts to monitor for signals, indicating unexpected benefits or adverse reactions. These signals provide valuable real-world intelligence that simply cannot be found by mining data from controlled clinical situations. Similarly, NLG technology can be applied to generate reports, or at least a framework, with human experts freed up to provide further analysis and polishing.
- Other use cases of AI in PV include auto-coding of terms used by consumers and non-medical personnel to official medical ontologies. The automatic converting of ADRs recorded by audio or video into text is another use case.
All of the reasons mentioned above explain why many companies should transition to proactive (and even predictive) risk management using AI tools. These technologies are already transforming the way PV tasks are performed. There is no doubt that with time, these tools will be advanced and improved to not only report AE but resolve challenges related to drugs for industry and health authorities.
Disclaimer: The insights and analysis in this article are the thoughts and opinions of the authored contributor with his colleague Hemant Sharma