Hybrid AI applications could be the future of pharmaceutical innovation
Christopher McKenna, Global Head of Professional Services and Consulting at Clarivate Analytics, offers his insight on the core topics of AI adoption and R&D productivity
Add bookmark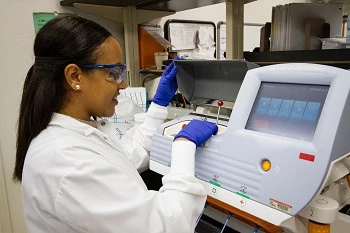
At this year’s JP Morgan Healthcare conference, technology remained a key focus for the pharmaceutical industry. Over a breakfast briefing, Christopher McKenna, Global Head of Professional Services and Consulting at Clarivate Analytics, offered insight on whether the hype around artificial intelligence (AI) is set to deliver a tangible impact on research and development.
In this exclusive interview with Pharma IQ, McKenna reviews current adoption levels, discusses the factors behind a successful AI project and explains why hybrid techniques could offer new insights.
Pharma IQ: With interest in AI continuing to grow in the pharmaceutical industry, what is the current level of adoption?
Christopher McKenna: When I think about where we are right now in the adoption of AI in pharma, the term irrational exuberance comes to mind.
We’re certainly at new heights in the hype cycle. Almost every new project we are starting in pharmaceuticals has some element of AI, machine learning (ML) or natural language processing (NLP) associated with it. It’s almost as ubiquitous as computing at this stage.
We’re definitely seeing certain applications further along than others. For example, some areas which have been more advanced computationally, such as computational chemistry and high-content screening, are more readily adopting deep neural networks, ML and different types of classification techniques. Further downstream, in clinical and regulatory, there is a growing willingness to use AI to improve processes, reduce cost and minimize risk, but the skill set needed to do so is not as present.
One area to always consider to grasp the adoption level of any technology in pharmaceuticals is the actions of regulatory bodies. What’s interesting is that regulators are certainly embracing AI and providing pathways for use in therapies, diagnostics and sensors, as can be seen by the exponential growing number of FDA approvals since 2015.
Pharma IQ: Many believe that AI will be the solution to the productivity problem in R&D. What impact do you see the technology having?
McKenna: The pharmaceutical industry has a productivity cloud hanging over it.
In the early 1990s, a paper was published by the head of research at Roche on the innovation deficit in pharmaceutical research. Since then, there has been a steady decline in the rate of drug approvals. In the past five years, however, we have seen a reversal of this innovation deficit with drug approvals increasing each year and accelerated approval pathways on the regulatory front providing new incentives to innovate.
But we’re still faced with an approximately 90 per cent failure rate for drugs going into Phase I.
When we look at AI and the role it can play in the industry, that’s the metric I’m really looking at: can this technology change our probability of success?
If you look at the use of AI in early research, from retro-synthetic planning, compound design and high content screening, it appears to be more of an incremental step using alternative computational techniques. These disciplines are readily adopting AI and building on computational techniques improved upon over decades, with AI representing an improvement on current methods.
I have seen AI having a far larger impact when you look at some of the applications in translational science and clinical development. At Clarivate, we run a number of projects using ML approaches to identify biomarkers, and then further use those biomarkers to stratify patients in clinical studies. With biomarker discovery and genomic medicine, you can really see how AI helps clinical researchers understand which patient is going to respond best to the drug. And because the clinical study can validate those predictions, the validation of the AI contribution is easily measured, although not always published.
“The pharmaceutical industry has a productivity cloud hanging over it.”
Christopher McKenna, Global Head of Professional Services and Consulting at Clarivate Analytics
Pharma IQ: What must a company keep in mind to ensure the success of its AI project?
McKenna: It really comes down to having a clear project goal for the AI system to work on.
It’s not about producing the algorithm. The modelling and algorithm part is relatively easy to do, but collecting the data, formatting, cleaning and determining the gold standard is the much slower part of the process. As highly structured data is critical to success, it is important to get this process right.
I would also recommend taking the time to look at the actual data available and explore which method of AI is going to best fit the problem at hand. It is not always about using the latest and greatest deep neural network.
For a project we ran on Crohn’s disease, we evaluated more than 50 different models before we selected an elasticnet method. This gave us time to fully structure the data and select a method that was the most predictive for the task at hand.
Pharma IQ: Looking forward, what will the industry need to consider next to fully benefit from the impact of AI and ML?
McKenna: In the pharmaceutical industry, the disciplines of chemistry, biology, clinical pharmacology, regulatory and marketing are dispersed. They talk different languages, approach problems in different ways and lack a single process. This is coupled with highly complex data sets. To accelerate the impact of AI, we need platforms to manage and automate different AI tools and bring this all together.
Two years ago, I had the opportunity to complete a patent landscape on machine learning. The one thing that really jumped out is that when you look at other industries, they’re often using multiple hybrid techniques in AI initiatives. Such hybrid techniques could bridge the gaps between different disciplines and offer new ways to bring together and analyze complex data.
It’s not just about using AI in small pockets, but running it across the business to implement learnings on a much larger scale. Imagine connecting de novo compound design to biological activity and toxicity prediction, to patent freedom to operate analysis, to indication prioritization, to success rate and cycle time prediction, and to market sizing, patient stratification and rNPV calculations. We are doing each of these alone in siloed projects today. Imagine when we connect these dots and have AI operating across the R&D value chain using hybrid approaches.