Reducing the drug discovery life cycle with AI
Pharma IQ speaks to Christos Nicolaou at Recursion Pharmaceuticals about reducing the drug discovery life cycle with artificial intelligence
Add bookmark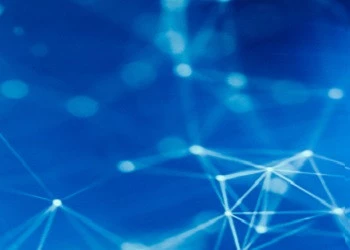
Ahead of Pharma IQ Live’s session Transforming drug discovery through artificial intelligence, Christos Nicolaou, PhD, Director in Digital Chemistry at Recursion Pharmaceuticals, tells us about reducing the drug discovery life cycle by using artificial intelligence (AI) and machine learning (ML) models.
Pharma IQ: How has AI changed the way you work?
Christos Nicolaou: Everything we do is data-driven, which is true for machines as well as for humans as we learn based on past examples and experiences. We have been trying to mimic this behavior for a long time using statistics and algorithms, but now we are at a stage where we have enough data and computational power to really take advantage of these things and surpass the knowledge we, as humans, are able to extract.
Right now, for example, the ability of an AI-driven algorithm to analyze billions of cell images and identify whether there has been a favorable interaction between the ligand and the cell during phenotypic screening, is something that no human can do. AI can do this pretty accurately.
Similarly, we can build models that capture all that information that is related to biological endpoints of interest, such as pharmacokinetics, e.g. absorption, distribution, metabolism and excretion (ADME), and provide insights and predictions never seen before. We have varying degrees of confidence, of course, but it is surprisingly accurate.
Pharma IQ: How do you measure this and ensure it is more accurate than what a human is capable of?
CN: I think the facts speak for themselves. In this industry one of the best things is that we continue theoretical research and silico predictions in the lab. As we design compounds and then make inferences using these AI models, we take those compounds, synthesise them and take them through experimental testing. That is where we are seeing improvements. When we look at the algorithms, each one of these models comes with a confidence measure and with accuracy statements.
Pharma IQ: Are there any areas you are particularly excited about that you think AI will transform next?
CN: In my opinion the greatest breakthroughs take place at the interaction between different fields of science. A very active domain of research has been the interaction between algorithms, data and automation. There are a number of players actively working on teaching or developing AI methodologies that identify synthetic routes for compounds of interest and prepare appropriate machine language instructions in order to guide automated synthesis robots. That synthesis is going to be done in a much faster way.
Within the framework of drug discovery, compound design will be associated with a specific route and that is not just going to be theoretical; it is also going to be instantiated in synthetic robot machine language within a workflow that will enable the creation of that chemical structure and for it to be sent for testing.
This is really exciting because it is going to allow us to essentially alleviate the human expert workload by passing on the more mundane tasks to the machines. In this manner the human will be able to do what it does best – which is create and design.
Pharma IQ: Will this speed up the process of drug discovery?
CN: Yes, that is our hope. Currently it takes months from the time that a molecule is designed, typically in silico, to send it off for synthesis where expert synthetic chemists will have to come up with a route, order the reagents and perform the reaction before purifying it, making sure the synthesis effort worked, and then passing it on for testing. Imagine having all these done under one roof executed by an automated platform. The goal is to go from months to weeks to days.
Pharma IQ: In terms of the work Recursion does, which areas are currently driving the use of AI in drug discovery?
CN: For the last few years, Recursion has been trying to decode biology. Recursion’s core business up to now has been to build maps of biology, the phenomaps, and use them to identify relations between all the potential mutations in genes and promising ligands. That is a huge undertaking as it creates gazillions of data points and potential relations. That is one area where AI can really help.
The other area is on the chemistry side. Pursuing ligands, developing them and optimizing them is anything but simple. We are in the process of building these chemistry capabilities and alongside several other companies, we have the goal of shrinking the time each discovery cycle takes, but also cutting the number of cycles down.
It is not just making things faster, it is also designing intelligently using all the AI/ML models that we can build, so that rather than make 10, 20 or 30 cycles we can get into the right kind of compounds in five. It is not only time saving, but also reduces cost.
Pharma IQ Live: Transforming drug discovery through artificial intelligence takes place on July 5-6. Catch Christos Nicolaou’s session on using AI and digital chemistry tools to accelerate drug discovery on July 5 at 12 pm EDT). Register for free here.