New workflow developed for mining epigenetic patterns
Add bookmark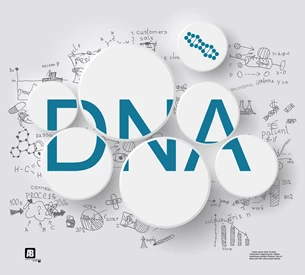
Dr Sheraz Gul of the Fraunhofer Institute for Molecular Biology discusses mining epigenetic patterns using age prediction algorithms.
The Human Genome Project resulted in the identification of a 2.91 billion base pair consensus sequence that related to the human genome [1] and subsequent research led to major advances in understanding human biology such as linking specific genes with diseases and the development of genetic tests.
Furthermore, it has been shown that genes can be silenced by epigenetic modifications, with DNA methylation being the most broadly studied and well-characterized. It is now known that the relationship between epigenetic changes are linked to a variety of disorders including cancer and immune disorders.
The Challenge
A number of studies have revealed that changes in epigenetics such as histone post translational modification and DNA methylation accompanies ageing [2]. Consequently, lifespan appears to be determined by epigenetics in addition to genetics and therefore it may be possible to alter lifespan by altering epigenetic information. DNA methylation is one of the most extensively studied epigenetic modifications during ageing such that in young cells, the majority of CpGs within the genome have cytosine methylation and when this occurs in promoters, it leads to transcriptional repression through the formation of compact chromatin structures. These modifications are controlled by the DNA methyltransferase family of enzymes and their modulation may open an avenue in reversing the epigenetic changes associated with ageing.
This has also given rise to the concept of an internal clock that is related to epigenetic methylation of specific sites in the genome which can be used to predict the biological age of individuals [3].
Want more? THE MYSTERIES OF ORBITAL EPIGENETICS AND CANCER RESEARCH
The data analysis workflow
Based on a tutorial and available data [4], we designed a workflow with the KNIME® Analytics Platform. Our workflow aims to be a highly configurable tool for analysing methylation data from different samples and to predict the biological age based on the methylation patterns discovered. We have expanded the workflow and implemented machine/deep learning algorithms to improve the prediction and recognition of methyl patterns and how they correlate with age.
Once robust and reliable algorithms have been developed we anticipate its exploitation in the form of a Genetic Age Test kit.
References:
[1] Venter JC, et al. The sequence of the human genome. Science, 2001, 291, 1304-51.
[2] Pal S. and Tyler JK. Epigenetics and aging. Sci. Adv., 2016, 2, e1600584.
[3] Wagner W. Epigenetic aging clocks in mice and men. Genome Biology, 2017, 18, 107.
[4] Horvath S. DNA methylation age of human tissues and cell types. Genome Biol. 2013, 14, R115.
This article was co-authored with Laura Chachulski and Andrea Zaliani who are both Bio/Chemoinformatics experts at the Fraunhofer IME-SP.